This website uses cookies so that we can provide you with the best user experience possible. Cookie information is stored in your browser and performs functions such as recognising you when you return to our website and helping our team to understand which sections of the website you find most interesting and useful.
Leveraging Transactional Data for Credit Risk Modeling
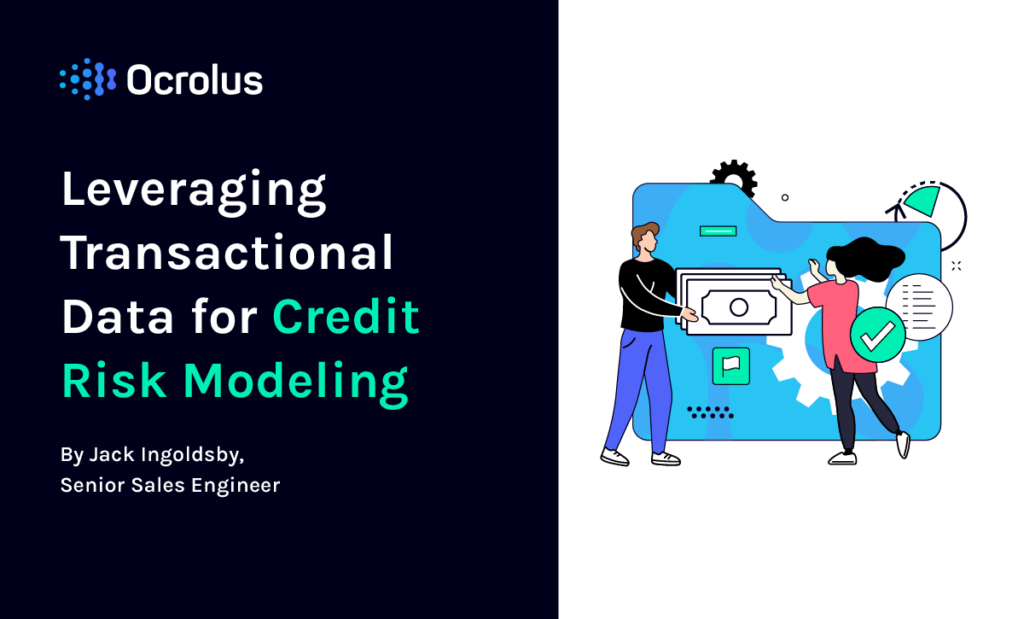
Lenders are looking for ways to automate document-driven lending processes to make them more efficient. Doing so allows them to accurately process more loan applications in less time, while providing a better experience for their customers. However, lenders might not be aware that they can also use automation for credit scoring in credit risk modeling. Accurate credit risk modeling is essential for understanding how likely a particular loan is to be repaid and, in turn, how that repayment or default will affect the lender’s own financial position.
Here’s a look at the current state of credit risk modeling, how lenders are leveraging automation to improve their credit models, and why high-quality transactional data is integral to the process of credit modeling.
The state of credit risk modeling today
For 21% of financial institutions surveyed, it can take more than four months to build, validate and deploy a new model, according to Cornerstone Advisors’ research report Credit Modeling and the Need for Speed. More than half of financial institutions feel they are ineffective at identifying origination opportunities they wouldn’t have otherwise taken—a finding that indicates lenders are concerned about meeting market growth goals.
Coming out of the COVID-19 crisis, many financial institutions are accelerating their credit model review cycles. The firms currently reviewing models every month expect to keep doing so, while nearly four in 10 financial institutions that review their credit models every quarter plan to shift to a monthly cycle. Half of the financial institutions with a semi-annual review expect to ramp up to a quarterly schedule in the future. These findings show that lenders increasingly see frequent credit risk modeling as a valuable tool for minimizing financial losses and maximizing their revenue opportunities.
How automation enables credit risk modeling
Automation can play a critical role in enabling the credit risk modeling process, helping lenders to quickly develop accurate and sophisticated models. Lenders are already using machine learning to create a more complete applicant profile and, from there, more accurately assess an applicant’s credit eligibility and risk of default.
With more comprehensive insight and better credit scoring, lenders can confidently issue more approvals while reducing loss rates. They can also use historical data from completed loans to experiment with models that improve pricing and reduce risk. These strategic capabilities enable lenders to grow their lending portfolios and maximize the value of their lending programs. They can also potentially aid in minimizing bias in lending and broadening access to credit.
To create these credit models, lenders either build their own custom solutions in-house or purchase pre-built solutions from vendors with extensive domain expertise. While the former option typically gives a lender maximum control over the design and execution of the solution, it requires a substantial investment of human capital and financial resources. The latter option relies on selecting an experienced vendor with deep knowledge of the lending space, but it usually allows a lender to get up and running more quickly at a lower cost.
Why credit risk modeling depends on high-quality data
Regardless of the credit modeling solution a lender chooses, high-quality transactional data is essential. However, accessing all of that data is not as simple as it may seem. Unstructured data is difficult to properly tag and analyze using an automation solution, leaving valuable insight on an applicant’s credit eligibility and risk untapped. Additionally, without a comprehensive applicant profile, even the most sophisticated credit model cannot help lenders make timely credit decisions at scale.
Here, too, automation can be beneficial. Solutions like Ocrolus can give lenders access to all of their data when creating a credit risk model. These kinds of solutions normalize unstructured data so it can be properly analyzed and then build a repeatable process for ingesting new data as it becomes available. This enables more accurate credit scoring going forward, ensuring that the lender can continue to create effective credit risk models even as circumstances change. With these capabilities, a lender can maintain and potentially increase its competitive advantage as the lending industry continues to digitally transform.
Leverage transactional data for credit risk modeling
AI is affecting the lending industry in multiple ways. Many lenders are already using automation to make their document-driven lending processes more efficient, which is a significant benefit from an operational and customer service perspective. They may not yet have considered how automation can also accelerate and improve credit risk modeling, yielding an even greater strategic advantage at a time when lenders are looking to continually update their credit models at a faster cadence.
High-quality transactional data is integral to the success of any credit modeling solution, and automation can also help lenders unlock it. By standardizing and cleaning their unstructured data so it can be analyzed and creating a regular process for ingesting the data, lenders can create accurate and up-to-date credit models on an ongoing basis. In doing so, they can grow their portfolios and gain a competitive advantage in a dynamic market.
Learn how Ocrolus’ automation solution unlocks lenders’ own data for use in credit risk modeling and beyond.