This website uses cookies so that we can provide you with the best user experience possible. Cookie information is stored in your browser and performs functions such as recognising you when you return to our website and helping our team to understand which sections of the website you find most interesting and useful.
Investor Insights: Why Data Approaching As Infrastructure Leads to “Owning the Pipes”
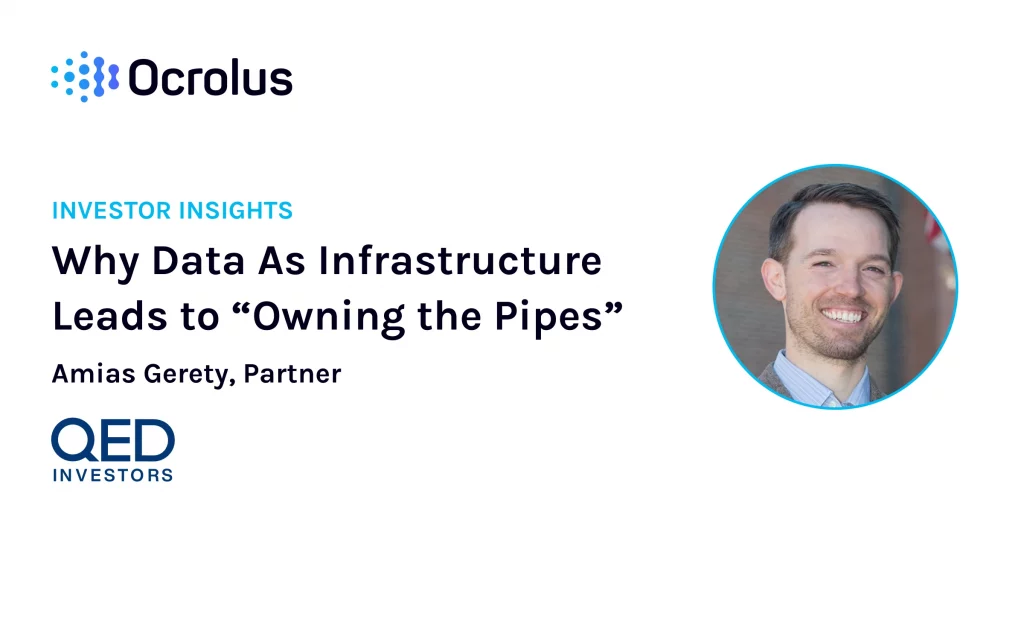
Today’s Investor Insights author, Amias Gerety, is a Partner at QED Investors. QED participated in Ocrolus’ Series A and B funding rounds. Amias joined QED in 2017 to support the fund’s portfolio and find new investment opportunities, with a focus on back-office technologies and infrastructure companies.
Amias offers a deep background in financial markets, compliance, and RegTech. Prior to his role at QED Investors, Amias served as Acting Assistant Secretary for Financial Institutions at the U.S. Department of the Treasury.
__________
All of the buzz around the benefits of data science reduces to a fundamental question of how to make good business decisions. And the challenge for data scientists is that most business decisions today are still made by humans, and in most well-run businesses, those decisions are actually pretty good.
Still, the human brain has some well understood limitations – it’s hard to scale, not good with large numbers, and gets tired easily. These limitations naturally lead to a lot of excitement about a new crop of startup software companies that can work all the time, at scale, and preserve most of the nuances of the human brain.
With this backdrop, naturally, I get a lot of pitches from companies bragging about the academic credentials of their data team or the patents that they’ve filed. Certainly, it seems kind of odd, in a world filled with the buzz of predictive analytics, neural networks, various forms of machine learning, and artificial intelligence, to argue that all this data science is not, in fact, an enduring source of value.
The practice of data science is the application of turning a model to a data set in order to inform or make a business decision. Abstracting from the specific technical choices, every vendor can take one of two approaches for the data and for the model they offer. For data, vendors can either aggregate the right data from public or purchased sources, or they can create proprietary data. For the code, they can either assemble the right code from well understood or “off the shelf” methods, or they can control some unique technical approach.
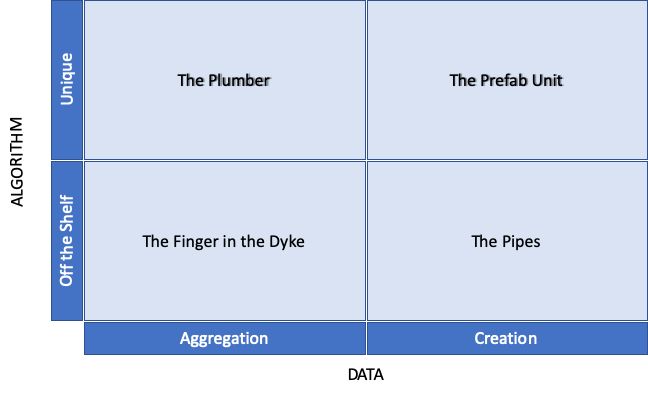
Let’s look at these choices with an analogy centering around water management, and let’s start in the top left of the grid above with the plumber. The distinction between aggregated and created data should be pretty straightforward, but bucketing a company’s approach to their models or their code requires a bit more explanation.
The Plumber
First, while there have been some real breakthroughs in data science approaches, as a non-technical investor I’m not particularly well-placed to assess whether a particular startup has achieved another breakthrough big enough to create a technical moat.
Secondly, even where a data science team has some unique capability – these teams still tend to operate like a plumber working on someone else’s data infrastructure. The plumber doesn’t own the problem — every client’s set up is just a little bit different. Moreover, the plumber can’t block any other plumbers from being called in to look at the problem.
The Finger in the Dyke
The plumber, however, is still far ahead of the little boy with his finger in the dyke. Companies that are based on using mostly open source machine learning models and applying them to public data sets and other people’s data are just like that little boy. The value is rushing against the dyke, not directed in any particular way, and no particular expertise is necessary to stop the water. The little boy can be a temporary hero, but it’s no way to act as a permanent solution.
The Prefab Unit
Now let’s go to the top right corner – this should be the “magic quadrant” approach — best data, best algorithm. What’s not to like?
I liken this quadrant to a pre-fab bathroom unit – the ones you see advertised on TV. For certain use cases, like those baths that have a door built in, the value prop seems to work. The plumbing and the design work together; the data and the algorithm are integrated. But for most startups, they simply don’t have enough data infrastructure or enough scale for truly proprietary approaches to become a significant differentiator.
Moreover, the ongoing rush of academic progress in the modeling realm will constantly put pressure on the clients to explore and experiment. Unless they can facilitate other internal or external teams viewing the data and testing competing modeling approaches, clients will view the product as a black box. Large corporations have data science teams that will demand transparency, and especially in regulated industries, expectations about explainability are implicitly embedded in legal standards.
The Pipes
Finally, we get to the bottom right data science approach – owning the pipes. At QED, we often say that sustainable competitive advantage comes from business processes that create proprietary data. For a software startup, getting into this category means embedding oneself deep in a client’s business processes, which will tend to increase lifetime value as a matter of course.
Just as importantly, operating in this space gives both the company and its clients optionality to get their modeling and their algorithms right over time. Rather than focus on building a unique modeling approach, the company can focus on testing many models to see where the data can help make better or faster business decisions.
Even the simplest bar charts and graphs have a tendency to add value if a data set is new. The most advanced machine learning won’t make a difference to decisions that are already made correctly. We encourage our companies to invest in their analytics groups for this reason, but also to be a facilitator of others. Following this path allows data to be treated as true infrastructure.
One of our most exciting companies is not only following this path towards data as infrastructure in its own business, but is also enabling financial services companies to follow this path themselves.
A forward-thinking data approach
Ocrolus extracts unstructured data from financial statements and structures the data to automate pre-underwriting and document verification steps for its lender customers. In doing so, it captures thousands of data points, that would otherwise flow through the system as data exhaust – never to be captured, analyzed, or understood. The data stream that Ocrolus analyzes for its lender customers, therefore, is purely proprietary. It’s a unique stream of unstructured data from each lender, representing the financial records of those who apply for loans.
Once Ocrolus and its customers have access to this proprietary data stream, the value that they can create even with basic application of new algorithmic approaches creates competitive advantage. Moreover, by delivering a proprietary data feed to its customers, Ocrolus allows its customers to build their own analytical value over time by delivering bespoke analytics, facilitating internal analytical teams, or even enabling other analytics vendors to add their proprietary view to a lender-customer.
Companies must remember that while data storage is now cheap, data gathering remains expensive. This is one of the core reasons that network effects emerge around data businesses. The rise of advanced analytics still hasn’t changed the old adage “garbage in, garbage out.” So both analytics vendors and their clients need to think carefully about what data they’re using, and separately, about what they’re doing with this data to make better business decisions. Doing so treats data as infrastructure and creates real value from proprietary data sources.
Learn more about what Ocrolus can do for your organization by booking your free demo.
Finding these insights helpful? Subscribe to our newsletter to stay up to date on forward-thinking trends and insights to help inform your business decisions.